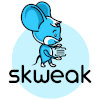
skweak
Example
GitHubNorskRegnesentral/skweak
Categories pipeline
standalone
research
training
Found a mistake or something isn't working?
If you've come across a universe project that isn't working or is incompatible with the reported spaCy version, let us know by opening a discussion thread.
Submit your project
If you have a project that you want the spaCy community to make use of, you can suggest it by submitting a pull request to the spaCy website repository. The Universe database is open-source and collected in a simple JSON file. For more details on the formats and available fields, see the documentation. Looking for inspiration your own spaCy plugin or extension? Check out the project idea section in Discussions.